- Generative AI Enterprise
- Posts
- Google VP on “36x API growth in 6 months”
Google VP on “36x API growth in 6 months”
Plus, Deloitte on scaling, Uber optimizing LLM training, and more.
WELCOME, EXECUTIVE AND PROFESSIONALS.
Since the last edition, we've reviewed hundreds of the latest insights on best practices, case studies, and innovation. Here’s the top 1%...
In today’s edition:
Google VP on “36x API growth in 6 months” across enterprises
How Uber optimizes in-house LLM training with open-source
Deloitte on scaling GenAI
Fast Fives: Transformation and technology in the news.
Career opportunities & events.
Read time: 4 minutes.

ENTERPRISE INSIGHT
Google VP on “36x API growth in 6 months”

Image source: Google
Brief: Burak Goktruk, VP of Engineering at Google Cloud AI & Industry Solutions, recently spoke as part of UC Berkeley’s “LLM Agents” course about the exponential rise in enterprise interest in generative AI. He pointed to “tens of thousands of enterprises calling and wanting to try” and a “36x growth in API usage in 6 months” from January, which “typically takes 5-10 years”.
Breakdown:
Enterprises are undergoing a "mindset change" with significantly less data required to use pre-trained large language models (LLMs) compared to legacy AI, which typically demanded extensive training datasets and iteration. This shift allows even non-specialist developers to create certain AI applications.
Most enterprises are focused on prompt engineering and retrieval-augmented generation (RAG), ensuring factual responses grounded in internal documents or the web.
Parameter-efficient fine-tuning methods like LoRA and QLoRA rather than full fine-tuning are gaining popularity for their lower computational needs.
As base models improve rapidly, enterprises often find that new model releases quickly outperform specialized, domain-specific versions.
Goktruk noted the growing use of sparse models, which reduce costs and improve latency by executing only a small fraction of pathways at inference time.
Choosing the right platform is key for most enterprises rather than specific models. API call costs are trending to zero due to improvements in GPU performance. Global demand for AI chips is "probably 100x" higher than supply.
Goktruk's 91 slides also cover topics such as the characteristics and benefits of common model adaptation approaches.
Why it’s important: The rapid pace of innovation in AI presents both opportunities and challenges for enterprises. To stay competitive, businesses must adapt by leveraging appropriate approaches and technologies.
CASE STUDY
How Uber optimizes in-house LLM training with open-source
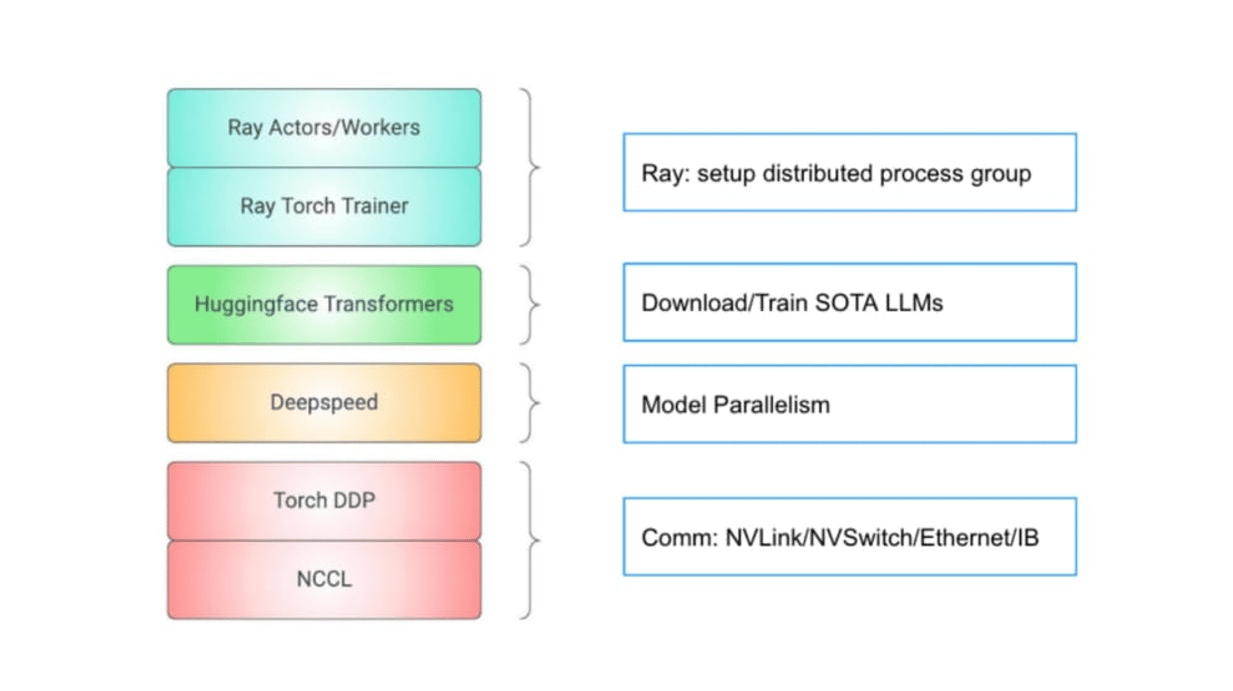
Image source: Uber
Brief: Uber's latest case study, featuring over 2,000 words and high-level architecture diagrams, explores how its in-house LLM training enhances flexibility, speed, and efficiency, using open-source models to help power generative AI-driven services.
Breakdown:
Uber leverages LLMs for Uber Eats recommendations, search, customer support chatbots, coding, and SQL query generation.
The case study details Uber’s infrastructure, training pipeline, and integration of open-source tools, libraries, and models to enhance LLM training.
Uber’s platform supports training the largest open source LLMs including full and parameter-efficient fine-tuning with LoRA and QLoRA.
Uber’s optimizations include CPU Offload and Flash Attention to achieve a 2-3x increase in throughput and a 50% reduction in memory usage.
Open-source models like Falcon, Llama, and Mixtral, along with tools like Hugging Face Transformers and DeepSpeed, enable Uber to adapt quickly.
Uber’s Ray and Kubernetes stack allows rapid integration of new open-source solutions, allowing for faster implementation.
Why it’s important: Uber demonstrates how open-source innovation, combined with robust infrastructure, can improve LLM training, offering a model to help other enterprises to accelerate generative AI development and deployment.
BEST PRACTICE INSIGHT
Deloitte on scaling GenAI

Image source: Deloitte
Brief: With GenAI use cases starting to proliferate across enterprises, Deloitte's new 22-slide report dives deeper into 13 key elements for scaling Generative AI, expanding on a framework from its latest adoption survey.
Breakdown:
Deloitte defines scale as a system’s ability to manage a growing amount of work, with decreasing unit costs. For GenAI, scaling also means transitioning from experimentation to sustainable, secure implementation aligned with business goals.
Strategy: Establish a comprehensive vision with a top-down mandate, target high-impact, low-barrier use cases, and evolve with an ecosystem of both existing providers and new market entrants.
Process: Implement repeatable governance processes to standardize work, address risks, ensure data security across the GenAI lifecycle and implement an agile operating model/delivery methods.
Talent: Engage stakeholders with the GenAI vision, foster adoption through documented roles and processes, and balance talent acquisition with workforce upskilling.
Data & Technology: Prioritize flexible IT architecture for enhancements, adopt agile methods for continuous improvement, and ensure data capabilities support GenAI strategy while focusing on cost management and output accuracy.
Nine indicators suggest an enterprise is on the right track, including faster time-to-market, increased value realization, lower unit costs for new capabilities, greater reusability to reduce development efforts, and more.
Why it’s important: Leading practices, processes, and technologies continue to evolve. While change is inevitable, pursuing scaling elements today positions enterprises to unlock business value with GenAI.

Capgemini released an 80-slide report highlighting how generative AI will reshape roles and streamline organizational structures. The report is based on surveys from 2,500 professionals across 15 countries.
Deloitte Netherlands published a 20-page whitepaper exploring how knowledge graphs combined with generative AI can enhance value. It includes use cases, implementation approaches, and architectural designs.
IBM reported that insurance leaders agree that rapid adoption of generative AI is necessary to compete, but insurance customers express reservations.
Bain & Co. expanded its partnership with OpenAI to develop industry-specific AI solutions. PwC also signed a ChatGPT Enterprise deal.
AWS Generative AI Innovation Center teamed with SailPoint to build an AI coding assistant using Anthropic's Claude Sonnet on Amazon Bedrock, accelerating SaaS connector development. Solution breakdown and key lessons learned included.

Perplexity launched ‘Internal Knowledge Search’ for enterprises: “From the web to your workspace, Perplexity searches it all”. They’re also in funding talks to more than double their valuation to $8 billion.
OpenAI reported ChatGPT's web traffic hit 3.1 billion visits in September 2024, a 112% year-over-year (YoY) increase, making it the 11th most visited site globally. They also introduced Swarm, an open-source framework for multi-agent AI.
Google updated NotebookLM, adding features for guiding the AI hosts focus/expertise level and announcing a business pilot program. They also partnered with Kairos Power to build 7 small nuclear-power reactors for AI.
Meta is collaborating with Blumhouse and select filmmakers to test Movie Gen AI, refining the tech before its public release in 2025. Meta's Yann LeCun also dismissed existential AI warnings as “complete BS”.
Databricks strengthened its partnership with AWS to accelerate custom models using Databricks Mosaic AI on AWS.

CAREER OPPORTUNITIES
Microsoft - Director, AI Co-Innovation Labs
Google - GTM Lead, AI
Oracle - Head of AI, West Europe
EVENTS
Deloitte - Data as a Product in the Era of GenAI - October 24, 2024
Galileo - GenAI Productionize 2.0 - October 29, 2024
Gartner - Responsible and Gen AI - November 15, 2024

Please take a moment to complete this short feedback survey to help us improve.
All the best,

Lewis Walker
Found this valuable? Share with a colleague.
Received this email from someone else? Sign up here
Let's connect on LinkedIn